A K-Means++ Clustering Implementation for VTK
Doria, David
Rensselaer Polytechnic Institute
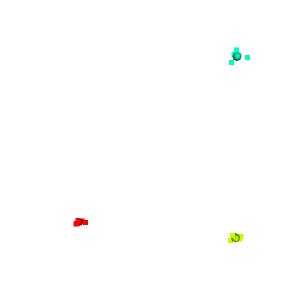
Please use this identifier to cite or link to this publication: http://hdl.handle.net/10380/3220
New: Prefer using the following doi: https://doi.org/10.54294/uv0hu9
Published in The VTK Journal - 2010 January - December Submissions.
Submitted by David Doria on 2010-09-28 12:51:44.
K-Means clustering is an excellent technique for clustering points when the number of clusters is known. We present a implementation (vtkKMeanClustering) of the algorithm written in a VTK context. We also implement the K-Means++ initialization method which finds the global optimum much more frequently than a naive/random initialization. The code is currently hosted at http://github.com/daviddoria/KMeansClustering .
BibTeX
One